MLflow
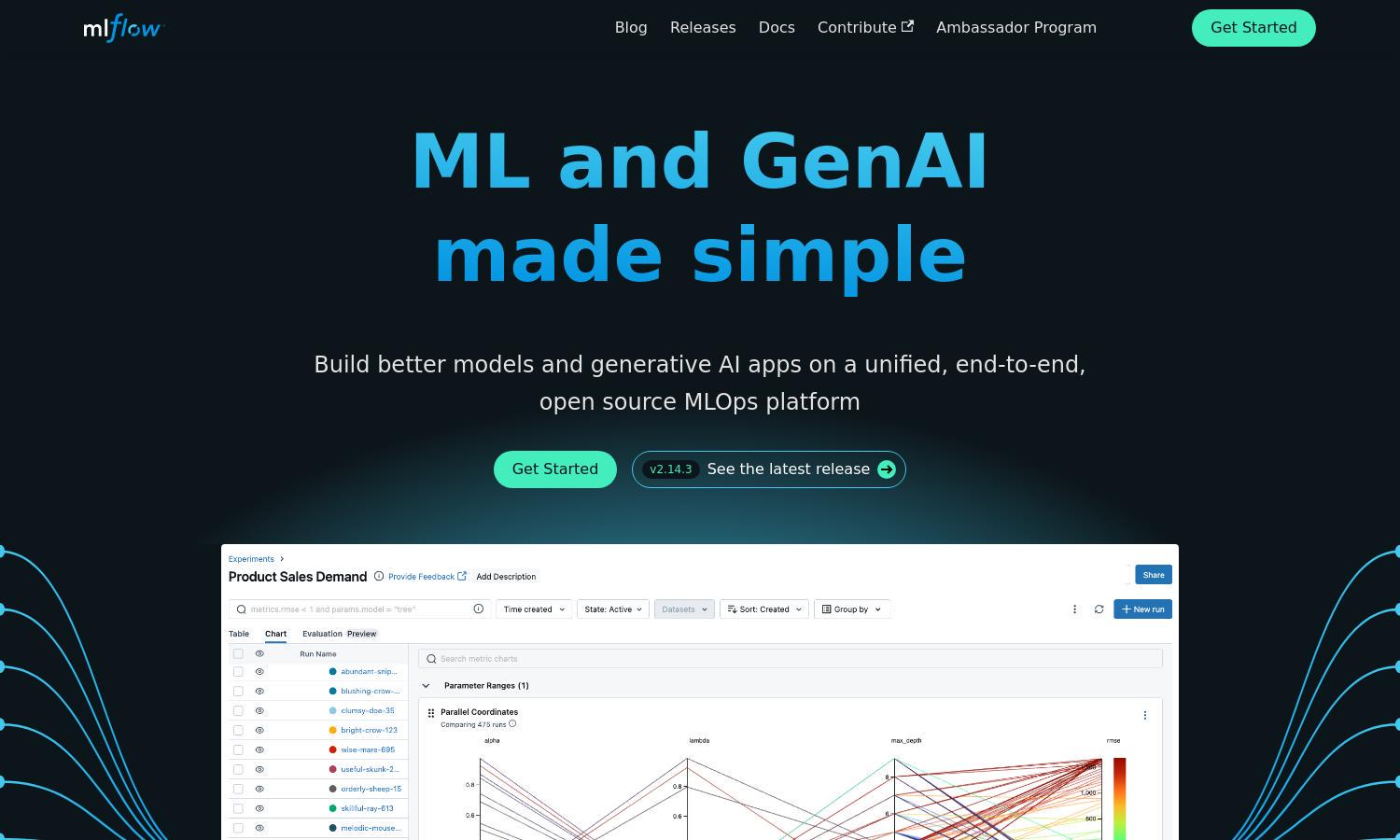
About MLflow
MLflow is an innovative open-source platform designed to simplify machine learning model management for data scientists and ML practitioners. It provides a seamless experience for tracking experiments, managing models, and deploying applications, enabling users to focus on building and optimizing ML and GenAI projects effectively.
MLflow offers free access with basic functionalities, while advanced features are available through annual subscriptions tailored for businesses. Pricing tiers provide various support levels and integrations to enhance productivity. Users benefit significantly from upgrading, gaining access to exclusive tools that improve model performance and collaboration.
MLflow's user interface is designed for intuitive navigation, allowing users to quickly track experiments and manage models. Its clean layout enhances the browsing experience by focusing on vital features. Users can easily access documentation and support materials, facilitating a smooth onboarding process and ensuring efficient use of the platform.
How MLflow works
Users begin with onboarding in MLflow by creating an account and accessing the dashboard. The platform allows them to log experiments, track parameters, and visualize results seamlessly. Users can then manage and deploy models while utilizing the various integrations that enhance their workflow, ensuring an efficient experience with MLflow.
Key Features for MLflow
Experiment Tracking
MLflow excels in experiment tracking, enabling users to log parameters and metrics efficiently. This unique feature helps data scientists to monitor their model's performance and make informed decisions, fostering better collaboration and optimization of machine learning projects throughout the entire lifecycle.
Model Registry
The Model Registry in MLflow allows users to catalog and manage models systematically. This key feature enhances tracking, versioning, and collaboration on models, ensuring teams have easy access to the latest versions and insights, ultimately leading to better deployment strategies and improved outcomes in machine learning projects.
Integrations with Popular Libraries
MLflow integrates seamlessly with numerous popular libraries such as TensorFlow, PyTorch, and Keras. This key feature provides users with the flexibility to enhance their machine learning models using their preferred tools, boosting productivity and ensuring compatibility with existing workflows across different platforms.
You may also like:
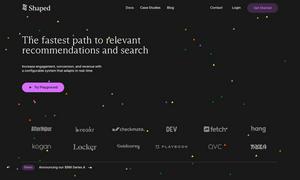